比赛记录
比赛记录
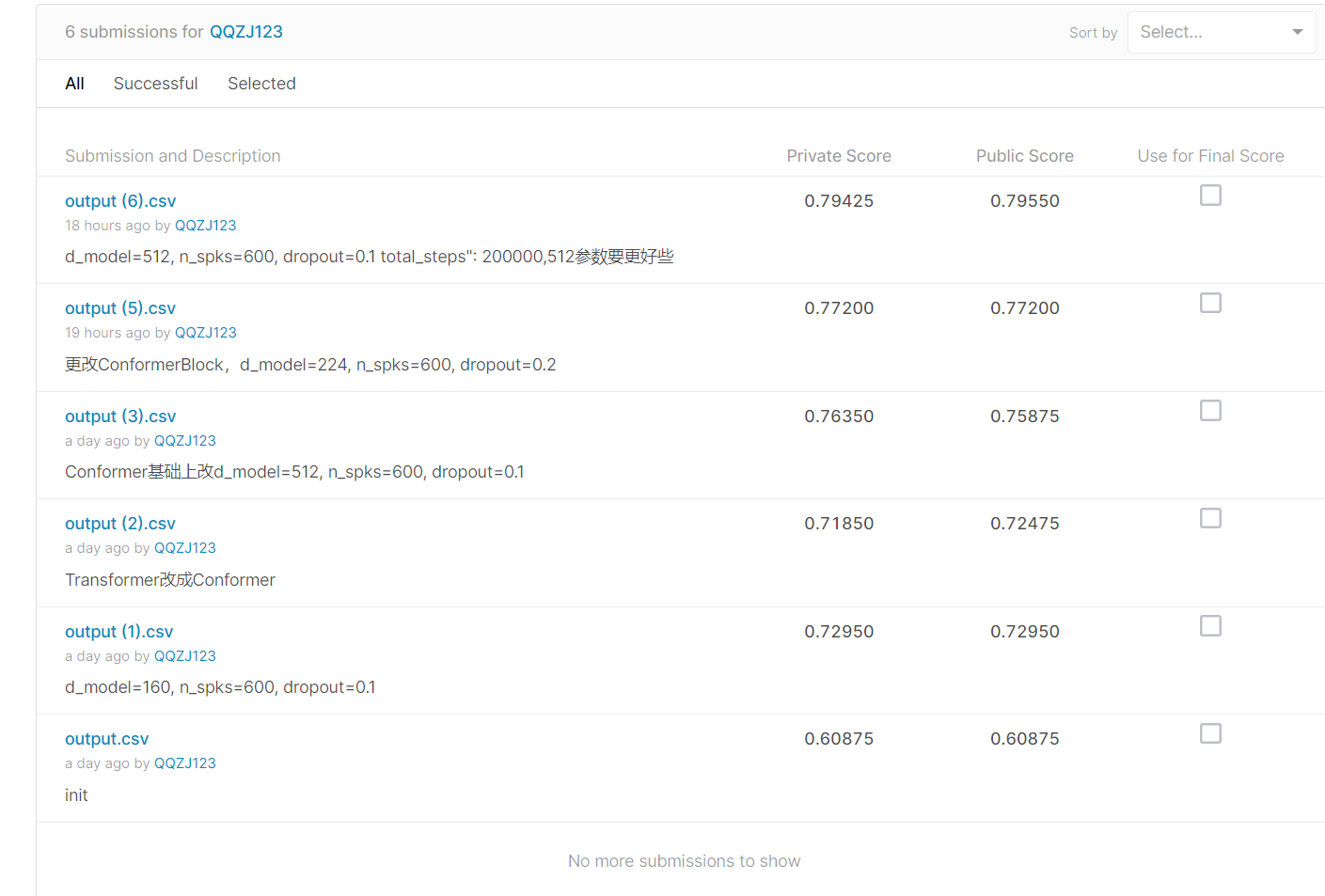
初始分数 0.60875
Conformer d_model=80, n_spks=600, dropout=0.1
第一次修改 0.72950
更改模型参数 d_model=160, n_spks=600, dropout=0.1 使用TransformerEncoder层 self.encoder = nn.TransformerEncoder(self.encoder_layer, num_layers=2)
第二次修改 0.71850
Transformer改成Conformer 发现结果反而变低了。 conformer的思路很简单,就是将Transformer和CNN进行结合。原因: 1.Transformer中由于attention机制,拥有很好的全局性。 2.CNN拥有较好的局部性,可以对细粒度的信息进行提取。 两者结合在语音上有较好的效果。论文中阐述了具体的model架构。
self.conformer_block=ConformerBlock(
dim=d_model,
dim_head=64,
heads=8,
ff_mult=4,
conv_expansion_factor=2,
conv_kernel_size=31,
attn_dropout=dropout,
ff_dropout=dropout,
conv_dropout=dropout
)
第三次修改 0.76350
Conformer基础上改d_model=512, n_spks=600, dropout=0.1 分数显著提高,看到文章说d_model不宜过高也不易过低
第四次修改 0.77200
论文:Conformer: Convolution-augmented Transformer for Speech Recognition transoformerEncoder改为ConformerBlock,d_model=224, n_spks=600, dropout=0.2 分数提高一点,推测更改ConformerBlock有效
self.encoder = ConformerBlock(
dim = d_model,
dim_head = 4,
heads = 4,
ff_mult = 4,
conv_expansion_factor = 2,
conv_kernel_size = 20,
attn_dropout = dropout,
ff_dropout = dropout,
conv_dropout = dropout,
)
第五次修改 0.79425
修改参数并且增加训练次数 d_model=512, n_spks=600, dropout=0.1 total_steps": 200000 推测最佳d_model在224--512之间要好些
第六次修改
更改维度 ConformerBlock self_Attentive_pooling AMsoftmax self_Attentive_pooling对于每一个特征,根据他自己的特征算出该给他赋予的权重。 amsoftmax的功能是让类内的特征靠的更近,类外的离得更远。
class AMSoftmax(nn.Module):
def __init__(self, in_feats, n_classes, m=0.3, s=15, annealing=False):
super(AMSoftmax, self).__init__()
self.linaer = nn.Linear(in_feats, n_classes, bias=False)
self.m = m
self.s = s
def _am_logsumexp(self, logits):
max_x = torch.max(logits, dim=-1)[0].unsqueeze(-1)
term1 = (self.s*(logits - (max_x + self.m))).exp()
term2 = (self.s * (logits - max_x)).exp().sum(-1).unsqueeze(-1) - (self.s*(logits-max_x)).exp()
return self.s * max_x + (term2 + term1).log()
def forward(self, *inputs):
x_vector = F.normalize(inputs[0], p=2, dim=-1)
self.linaer.weight.data = F.normalize(self.linaer.weight.data, p=2,dim=-1)
logits = self.linaer(x_vector)
scaled_logits = (logits-self.m)*self.s
return scaled_logits - self._am_logsumexp(logits)
class self_Attentive_pooling(nn.Module):
def __init__(self, dim):
super(self_Attentive_pooling, self).__init__()
self.sap_linaer = nn.Linear(dim, dim)
self.attention = nn.Parameter(torch.FloatTensor(dim,1))
torch.nn.init.normal_(self.attention, std=.02)
print(1)
def forward(self, x):
# x = x.permute(0, 2, 1)
h = torch.tanh(self.sap_linaer(x))
w = torch.matmul(h, self.attention).squeeze(dim=2)
w = F.softmax(w, dim=1).view(x.size(0), x.size(1), 1)
x = torch.sum(x * w, dim=1)
return x